Report 2: The Digital Image / Image Processing
MAT255 Techniques, History & Aesthetics of the Computational Photographic Image
https://www.mat.ucsb.edu/~g.legrady/aca ... s255b.html
Please provide a response to any of the material covered in this week's two presentations by clicking on "Post Reply". Consider this to be a journal to be viewed by class members. The idea is to share thoughts, other information through links, anything that may be of interest to you and the topic at hand.
Report for this topic is due by April 22, 2022 but each of your reports can be updated throughout the length of the course.
Report 2: The Digital Image / Image Processing
-
- Posts: 8
- Joined: Tue Mar 29, 2022 3:33 pm
Re: Report 2: The Digital Image / Image Processing
One topic from the past two weeks of class that caught my attention was the concept of metadata. Specifically, a major difference between analog and digital photography is that digital photography captures metadata while analog photography does not. This concept stuck out to me for two reasons. First, I was curious about the history of metadata in digital images. Specifically, why do images capture the information that they do (and not other information)? Second, there seems to be an inherent irony to a digital image’s metadata; the photographer captures an image through a digital device, but the image, in some ways, also captures the photographer through metadata. This is especially true for non-professional photographers who use digital cameras on their phones and upload this content to online platforms. Thus, in this report, I review a brief history of metadata in digital photography and explore one instance of the irony manifested through digital images’ metadata.
To review the history of image metadata, it must first be mentioned that metadata has always existed in images. For example, on the back of paintings, artists, exhibitions, and collectors have often written notes, placed labels, inscribed inventory numbers, and more. Similarly, analog photographs often contain this type of “metadata”. As a personal account, I have rifled through my family photo albums and seen labels or inscriptions on photos of the location and persons in them. This has been a common practice in many analog photographs along with other types of “metadata”.
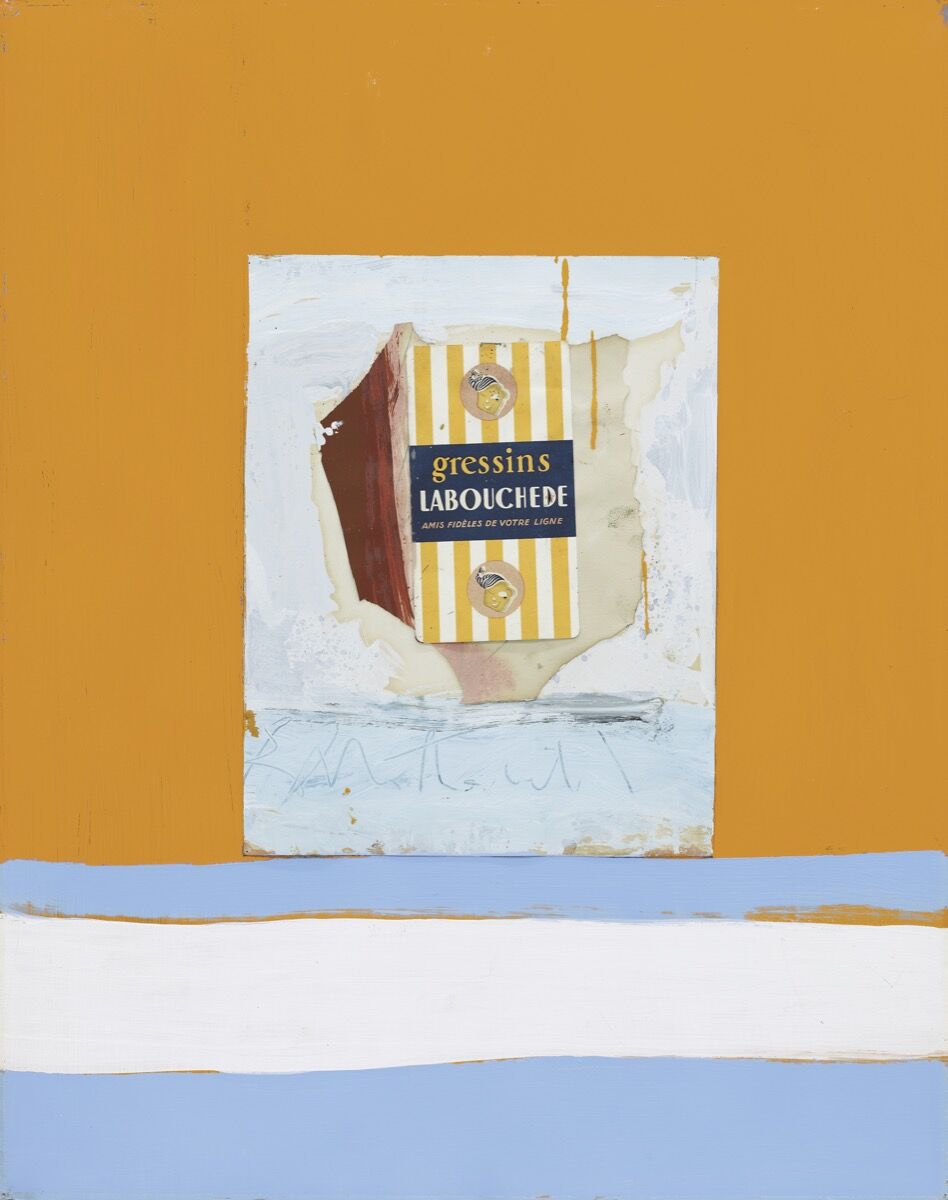
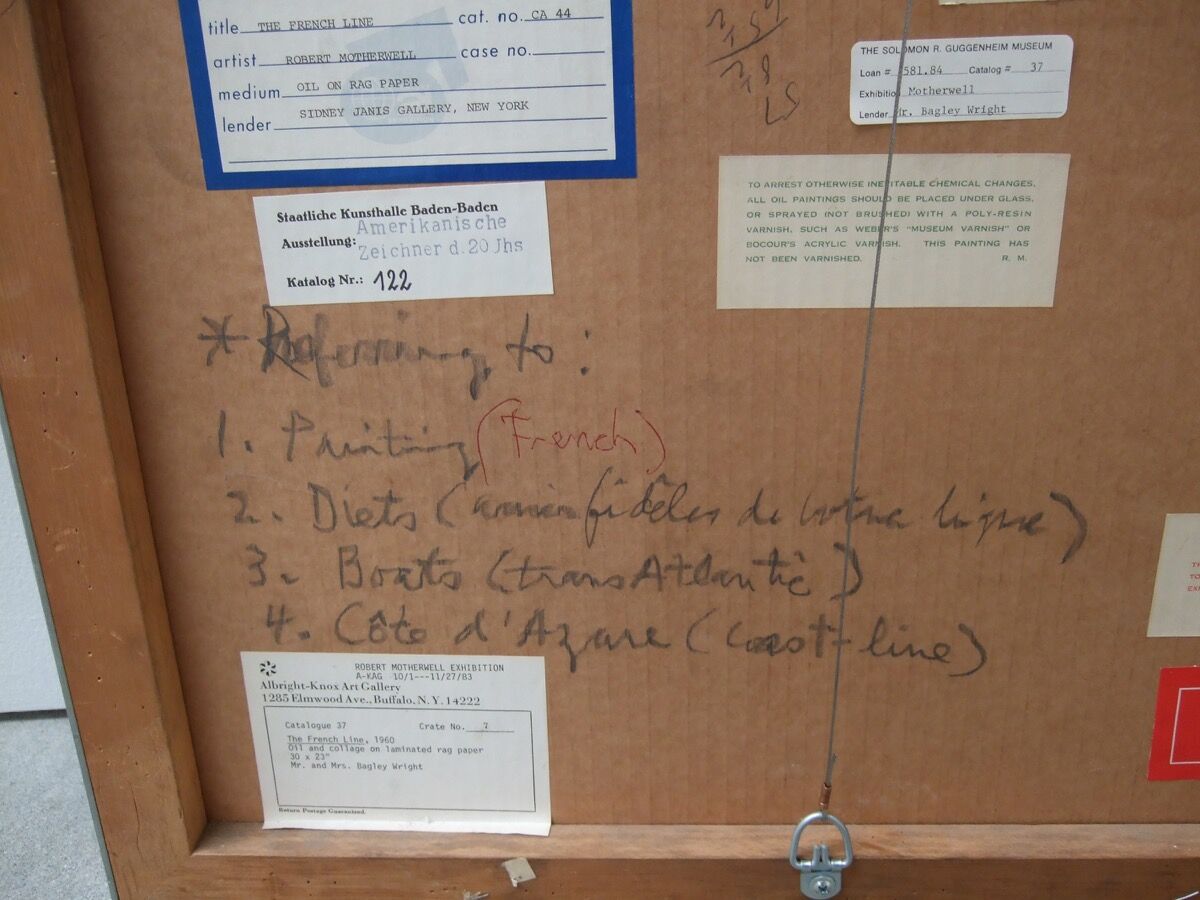
Front and back of Robert Motherwell, The French Line, 1960. Retrieved from https://www.artsy.net/article/artsy-edi ... s-artworks
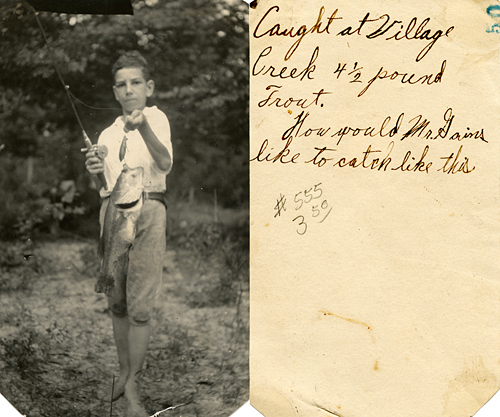
Thrifted photograph and writing on back shared by blogger from https://texasflycaster.com/old-fishing- ... -interest/
For digital images, the standardization of metadata as we know it can be attributed to the Japanese Camera Industry Association and the International Press Telecommunications Council (IPTC). In the 1990s, the Japanese Camera Industry Association created the exchangeable image file format (EXIF) to capture information about a digital camera’s settings, make and model, date and time, and GPS coordinates. This information is basic metadata that is now standard in every digital camera. Around the same time, the IPTC defined what standards and attributes should be included in images for more administrative purposes. The IPTC’s metadata includes information like the image’s creator, titles, captions, copyright, permissions, and licensing. EXIF and IPTC metadata were intended to help organize photographs, know how a certain photograph was captured, and standardize the dissemination of photographs between news outlets. However, as with many ideas, while intentions may have been focused in one direction, the entirety of the consequences cannot be foretold.
This brings me to the initial irony I mentioned. Because a digital camera automatically creates metadata, a person who thinks they are capturing a subject is also capturing information about themselves. As an example of the irony in context, I immediately think of big tech surveillance. Everyday billions of users upload images to social media platforms and unbeknownst to them hand over metadata in these photos as well. I list below example statements of privacy policies from social media platforms that I frequent:
Facebook/Instagram https://www.facebook.com/policy.php: To provide the Meta Products, we must process information about you. The type of information that we collect depends on how you use our Products. We collect the content, communications, and other information you provide when you use our Products, including when you sign up for an account, create or share content, and message or communicate with others. This can include information in or about the content that you provide (e.g. metadata), such as the location of a photo or the date a file was created.
TikTok https://www.tiktok.com/legal/privacy-policy-us?lang=en: We collect User Content through pre-loading at the time of creation, import, or upload, regardless of whether you choose to save or upload that User Content […] We may collect information about the images and audio that are a part of your User Content, such as identifying the objects and scenery that appear, the existence and location within an image of the face and body features and attributes, the nature of the audio, and the text of the words spoken in your User Content. We generally use the information we collect: to send promotional materials from us or on behalf of our affiliates and trusted third parties; to make suggestions and provide a customized ad experience; to facilitate sales, promotion, and purchases of goods and services and to provide user support.
Reddit https://www.redditinc.com/policies/priv ... er-12-2021: We collect the content you submit to the Services. Your content may include text, links, images, gifs, and videos […] we use this information about you to provide, maintain, and improve the services; research and develop new services; monitor and analyze trends, usage, and activities in connection with our Services; measure the effectiveness of ads shown on our Services, and personalize the Services, and provide and optimize advertisements, content, and features that match user profiles or interests.
Unsurprisingly, when a user uploads an image in any capacity, these platforms capture the information provided and use this data to their ends. Indeed, companies use vague phrases like “to provide Meta Products” or explicit phrases like “to facilitate sales, promotion, and purchases of goods and services”. So even though these privacy policies are publicly available, and users could be made aware of what happens to the information they upload, users do not have any control over the matter.
To emphasize the irony further, a subject of a photograph may not know that their image is being captured or might not know what their image will be used for. The photographer holds that power. However, a photographer displaying their image online can have a parallel experience. A photographer who uploads an image may not know how that image’s metadata is collected or what that metadata will be used for. The platform holds that power.
References:
To review the history of image metadata, it must first be mentioned that metadata has always existed in images. For example, on the back of paintings, artists, exhibitions, and collectors have often written notes, placed labels, inscribed inventory numbers, and more. Similarly, analog photographs often contain this type of “metadata”. As a personal account, I have rifled through my family photo albums and seen labels or inscriptions on photos of the location and persons in them. This has been a common practice in many analog photographs along with other types of “metadata”.
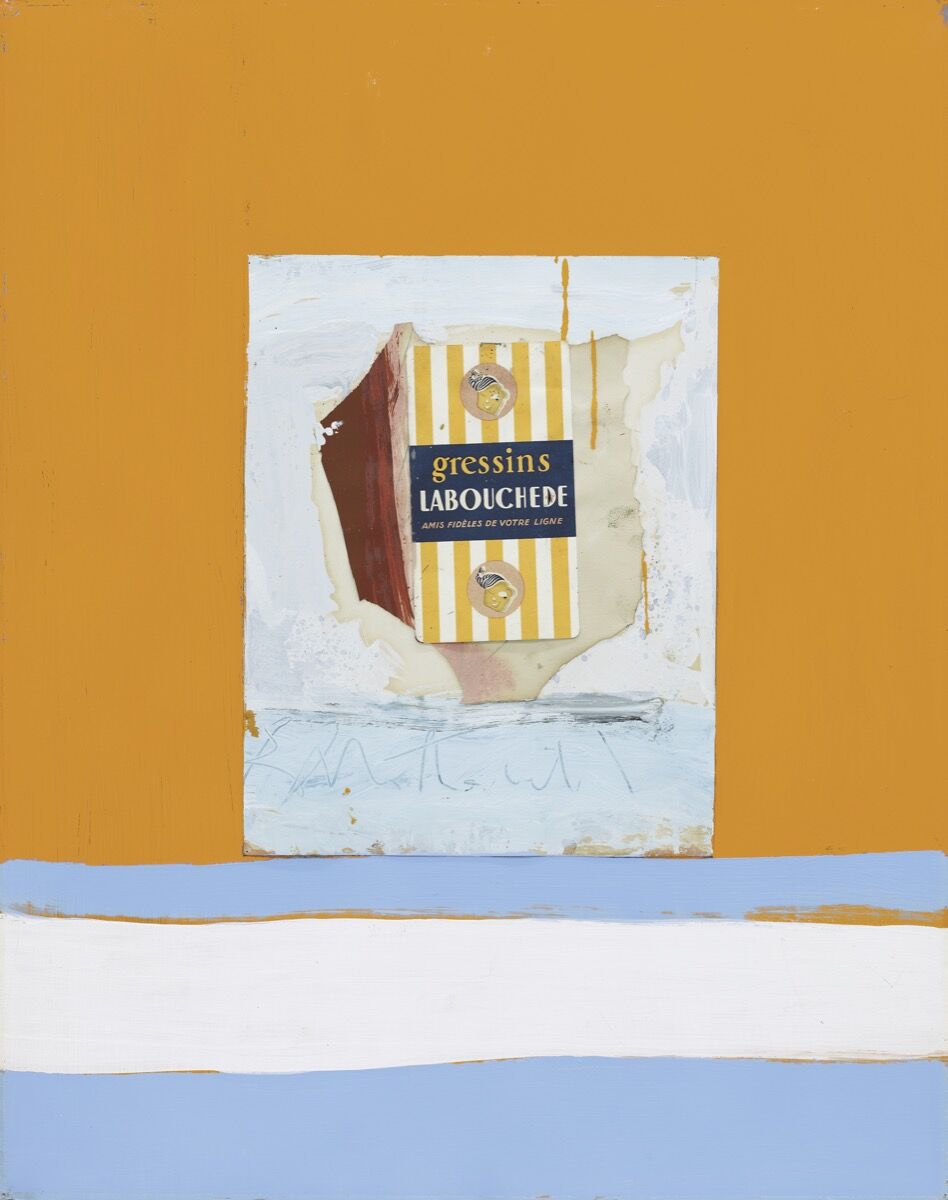
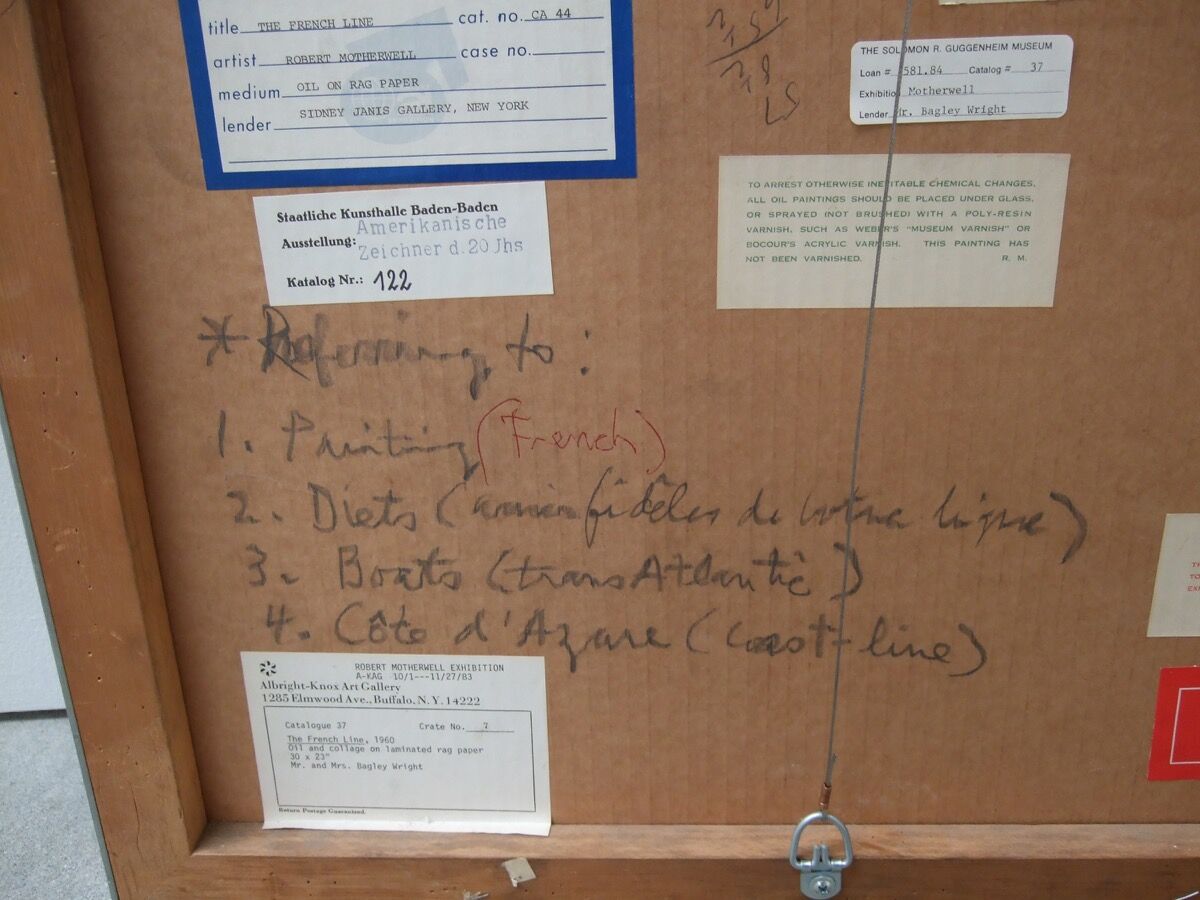
Front and back of Robert Motherwell, The French Line, 1960. Retrieved from https://www.artsy.net/article/artsy-edi ... s-artworks
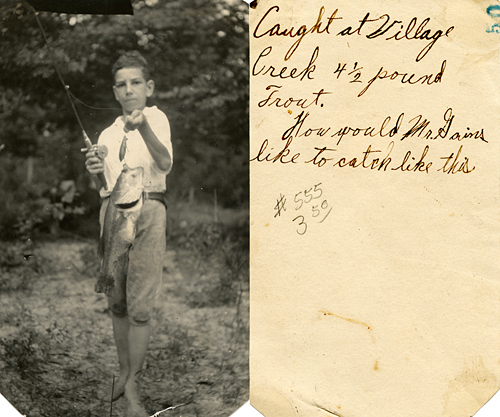
Thrifted photograph and writing on back shared by blogger from https://texasflycaster.com/old-fishing- ... -interest/
For digital images, the standardization of metadata as we know it can be attributed to the Japanese Camera Industry Association and the International Press Telecommunications Council (IPTC). In the 1990s, the Japanese Camera Industry Association created the exchangeable image file format (EXIF) to capture information about a digital camera’s settings, make and model, date and time, and GPS coordinates. This information is basic metadata that is now standard in every digital camera. Around the same time, the IPTC defined what standards and attributes should be included in images for more administrative purposes. The IPTC’s metadata includes information like the image’s creator, titles, captions, copyright, permissions, and licensing. EXIF and IPTC metadata were intended to help organize photographs, know how a certain photograph was captured, and standardize the dissemination of photographs between news outlets. However, as with many ideas, while intentions may have been focused in one direction, the entirety of the consequences cannot be foretold.
This brings me to the initial irony I mentioned. Because a digital camera automatically creates metadata, a person who thinks they are capturing a subject is also capturing information about themselves. As an example of the irony in context, I immediately think of big tech surveillance. Everyday billions of users upload images to social media platforms and unbeknownst to them hand over metadata in these photos as well. I list below example statements of privacy policies from social media platforms that I frequent:
Facebook/Instagram https://www.facebook.com/policy.php: To provide the Meta Products, we must process information about you. The type of information that we collect depends on how you use our Products. We collect the content, communications, and other information you provide when you use our Products, including when you sign up for an account, create or share content, and message or communicate with others. This can include information in or about the content that you provide (e.g. metadata), such as the location of a photo or the date a file was created.
TikTok https://www.tiktok.com/legal/privacy-policy-us?lang=en: We collect User Content through pre-loading at the time of creation, import, or upload, regardless of whether you choose to save or upload that User Content […] We may collect information about the images and audio that are a part of your User Content, such as identifying the objects and scenery that appear, the existence and location within an image of the face and body features and attributes, the nature of the audio, and the text of the words spoken in your User Content. We generally use the information we collect: to send promotional materials from us or on behalf of our affiliates and trusted third parties; to make suggestions and provide a customized ad experience; to facilitate sales, promotion, and purchases of goods and services and to provide user support.
Reddit https://www.redditinc.com/policies/priv ... er-12-2021: We collect the content you submit to the Services. Your content may include text, links, images, gifs, and videos […] we use this information about you to provide, maintain, and improve the services; research and develop new services; monitor and analyze trends, usage, and activities in connection with our Services; measure the effectiveness of ads shown on our Services, and personalize the Services, and provide and optimize advertisements, content, and features that match user profiles or interests.
Unsurprisingly, when a user uploads an image in any capacity, these platforms capture the information provided and use this data to their ends. Indeed, companies use vague phrases like “to provide Meta Products” or explicit phrases like “to facilitate sales, promotion, and purchases of goods and services”. So even though these privacy policies are publicly available, and users could be made aware of what happens to the information they upload, users do not have any control over the matter.
To emphasize the irony further, a subject of a photograph may not know that their image is being captured or might not know what their image will be used for. The photographer holds that power. However, a photographer displaying their image online can have a parallel experience. A photographer who uploads an image may not know how that image’s metadata is collected or what that metadata will be used for. The platform holds that power.
References:
- Metadata History: Timeline | Photometadata.org. (n.d.). Retrieved April 6, 2022, from https://www.photometadata.org/META-Reso ... y-Timeline
- What is EXIF Data and How You Can Remove it From Your Photos. (2020, April 23). Retrieved April 6, 2022, from Photography Life website: https://photographylife.com/what-is-exif-data
- What is Photo Metadata. (n.d.). Retrieved April 6, 2022, from IPTC website: https://iptc.org/standards/photo-metada ... -metadata/
-
- Posts: 11
- Joined: Thu Jan 07, 2021 2:59 pm
Re: Report 2: The Digital Image / Image Processing
Talking about image metadata and steganography in this week’s lecture made me think about the security implications present by being able to embed data within an image.
Sienna brought up a good point in her post about how since cameras capture metadata automatically, even if the photo subject is not themselves, a person is revealing information about themselves anyway through the metadata. This reminded me of the point I made in my last week’s discussion about how images can be deceiving and what a viewer sees is not an accurate portrayal of what really is.
As mentioned in the lecture, steganography in recent times is the concealment of information within computer files. But steganography is not a new invention. Old steganographic techniques can be dated back to the 1400s, hiding text within other text. The table below is a table contained in Book 3 of “Steganographia” by Johannes, referencing text within the book. When decoded, the resulting message becomes clear to the reader[1]. A more well known instance of this can be seen in a clip from National Treasure, where Nicholas Cage uses lemon to reveal invisible ink (another form of steganography) written on the back of the Declaration of Independence. The resulting message is a series of numbers, referencing the text of other notable documents (again a form of steganography)[2].
As technology has advanced, steganography techniques have moved from physical to digital. One thing I didn’t know was possible was that images can be embedded into sound files. If these sound files are analyzed with a spectrogram, the image is revealed.
Here are a few examples of analyzing some songs and their resulting images:
More examples can be found here [3].
Messages can be hidden within images themselves too. As mentioned in the lecture, by making use of the last significant bit of pixel data, hidden messages can be concealed. And it’s not just text that can be concealed in an image. A whole other image can be hidden inside an image [4].
As we can see, the merged image looks identical to the original image to the naked eye. It is so crazy how a whole other image can be hidden within another image. This again illustrates the point about how an image can capture and show one thing but is not telling the whole story. Not only that, but this presents a lot of security vulnerabilities. What if a malicious sender conceals a virus within an image? With images today, we cannot just trust what we see to get the whole picture.
Works Cited
1. https://www.giac.org/paper/gsec/1714/st ... ure/101649
2. https://www.youtube.com/watch?v=WTA7KR9-9lM
3. https://twistedsifter.com/2013/01/hidde ... trographs/
4. https://towardsdatascience.com/steganog ... ca66b2acb1
Sienna brought up a good point in her post about how since cameras capture metadata automatically, even if the photo subject is not themselves, a person is revealing information about themselves anyway through the metadata. This reminded me of the point I made in my last week’s discussion about how images can be deceiving and what a viewer sees is not an accurate portrayal of what really is.
As mentioned in the lecture, steganography in recent times is the concealment of information within computer files. But steganography is not a new invention. Old steganographic techniques can be dated back to the 1400s, hiding text within other text. The table below is a table contained in Book 3 of “Steganographia” by Johannes, referencing text within the book. When decoded, the resulting message becomes clear to the reader[1]. A more well known instance of this can be seen in a clip from National Treasure, where Nicholas Cage uses lemon to reveal invisible ink (another form of steganography) written on the back of the Declaration of Independence. The resulting message is a series of numbers, referencing the text of other notable documents (again a form of steganography)[2].
As technology has advanced, steganography techniques have moved from physical to digital. One thing I didn’t know was possible was that images can be embedded into sound files. If these sound files are analyzed with a spectrogram, the image is revealed.
Here are a few examples of analyzing some songs and their resulting images:
More examples can be found here [3].
Messages can be hidden within images themselves too. As mentioned in the lecture, by making use of the last significant bit of pixel data, hidden messages can be concealed. And it’s not just text that can be concealed in an image. A whole other image can be hidden inside an image [4].
As we can see, the merged image looks identical to the original image to the naked eye. It is so crazy how a whole other image can be hidden within another image. This again illustrates the point about how an image can capture and show one thing but is not telling the whole story. Not only that, but this presents a lot of security vulnerabilities. What if a malicious sender conceals a virus within an image? With images today, we cannot just trust what we see to get the whole picture.
Works Cited
1. https://www.giac.org/paper/gsec/1714/st ... ure/101649
2. https://www.youtube.com/watch?v=WTA7KR9-9lM
3. https://twistedsifter.com/2013/01/hidde ... trographs/
4. https://towardsdatascience.com/steganog ... ca66b2acb1
-
- Posts: 15
- Joined: Tue Mar 29, 2022 3:30 pm
Re: Report 2: The Digital Image / Image Processing
During last week's lectures, we've briefly discussed over computer vision functions, such as object identification, pattern recognition, and motion tracking. Part of my research concerns studying and understanding the works of facial recognition and public surveillance. Now, though my research program touches upon these topics, its primary concern is how the advancement of technology affects people of color (POC) in a society that previously and currently ostracizes them. If humans have proven to show bias towards certain skin colors and racial features, it's vital to predict, detect, and understand how such bias persists in the machines they program.
In an article written by Nick Galov for the Web Tribunal, Galov writes closely about the accuracy of facial recognition software and its own racial bias:
Though facial/object recognition software bias may seem trivial in the scope of social media - such as Facebook mistakenly identifying and tagging you in a friend's photo - it can have more serious implications when accounting for government surveillance. A surprising fact for most people, approximately one in two Americans have their faces registered in a law enforcement facial recognition database [2]. I've attached an image below to show how many of these adults approximately approve or disprove of the use of facial and object identification software in the scope of big tech companies versus government agencies.
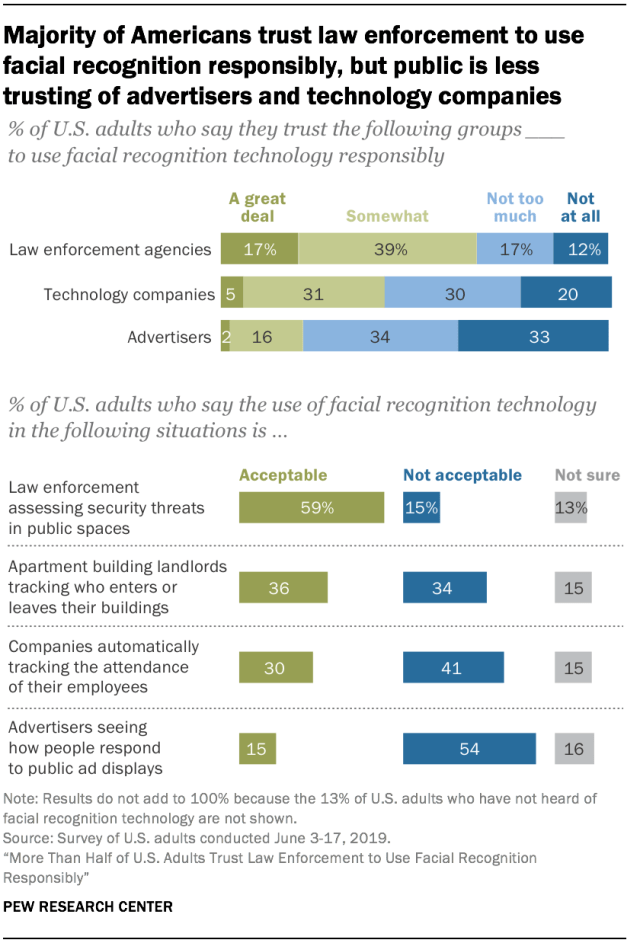
Though the overall consensus seems to be that people trust government agencies more than their big tech counterparts in terms of their privacy and overall ethics, understanding the specific implications for people of color could possibly yield different results. When identification errors become 35 times more prevalent when attempting to identify black females, then this same racial population can potentially be wrongly targeted and accused for the actions of another. One practical solution to this is having a larger array of people of color used in the programming process of such machinery. Through the machine learning process, having a more prominent focus on differentiating black, latino, and indigenous features amongst a larger plethora of individuals within these racial subgroups can help diminish further inaccuracies.
Overall, this remains as just one case in proving just how vital it is to be conscious of racial implications when programming the next technological advancement.
Sources:
[1] Galov, Nick. “20 Facial Recognition Statistics to Scan through in 2022.” WebTribunal, 8 Apr. 2022, https://webtribunal.net/blog/facial-rec ... tics/#gref.
[2] Najibi, Alex. “More than Half of U.S. Adults Trust Law Enforcement to Use Facial Recognition Responsibly.” Pew Research Center: Internet, Science
& Tech, Pew Research Center, 27 Aug. 2020, https://www.pewresearch.org/internet/20 ... sponsibly/.
In an article written by Nick Galov for the Web Tribunal, Galov writes closely about the accuracy of facial recognition software and its own racial bias:
FR systems can achieve up to 99.97% accuracy. Face-scanning stats indicate identification errors are 35 times more likely to happen to a black female compared to a white male [1].
Though facial/object recognition software bias may seem trivial in the scope of social media - such as Facebook mistakenly identifying and tagging you in a friend's photo - it can have more serious implications when accounting for government surveillance. A surprising fact for most people, approximately one in two Americans have their faces registered in a law enforcement facial recognition database [2]. I've attached an image below to show how many of these adults approximately approve or disprove of the use of facial and object identification software in the scope of big tech companies versus government agencies.
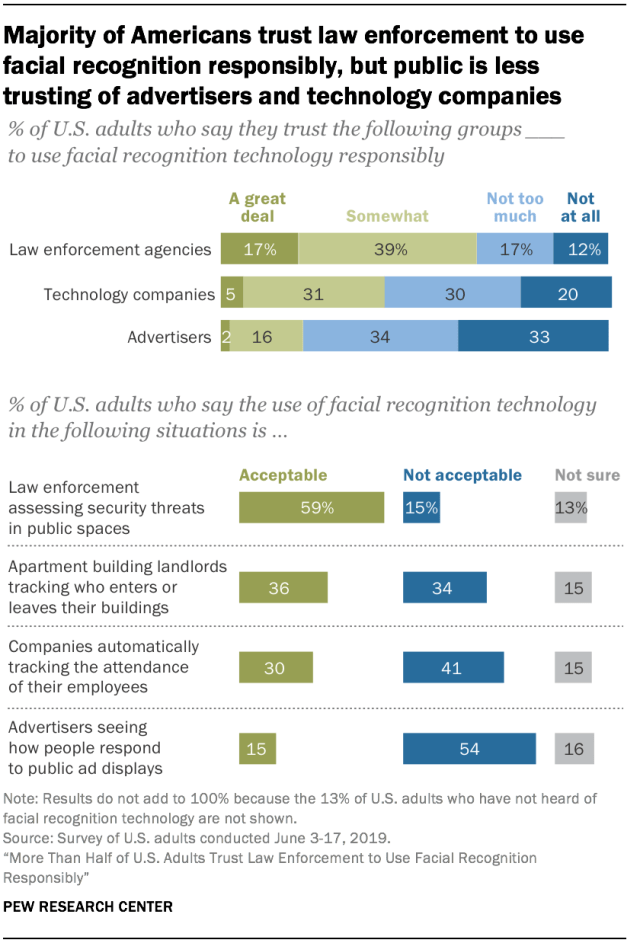
Though the overall consensus seems to be that people trust government agencies more than their big tech counterparts in terms of their privacy and overall ethics, understanding the specific implications for people of color could possibly yield different results. When identification errors become 35 times more prevalent when attempting to identify black females, then this same racial population can potentially be wrongly targeted and accused for the actions of another. One practical solution to this is having a larger array of people of color used in the programming process of such machinery. Through the machine learning process, having a more prominent focus on differentiating black, latino, and indigenous features amongst a larger plethora of individuals within these racial subgroups can help diminish further inaccuracies.
Overall, this remains as just one case in proving just how vital it is to be conscious of racial implications when programming the next technological advancement.
Sources:
[1] Galov, Nick. “20 Facial Recognition Statistics to Scan through in 2022.” WebTribunal, 8 Apr. 2022, https://webtribunal.net/blog/facial-rec ... tics/#gref.
[2] Najibi, Alex. “More than Half of U.S. Adults Trust Law Enforcement to Use Facial Recognition Responsibly.” Pew Research Center: Internet, Science
& Tech, Pew Research Center, 27 Aug. 2020, https://www.pewresearch.org/internet/20 ... sponsibly/.